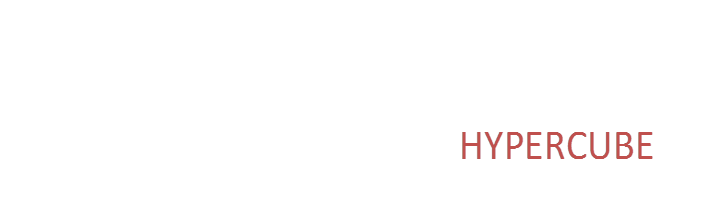
Python API v3.0
- Quickstart
- Connect
- Explore
- Main objects to explore
- Usage Examples
- Main API objects
- Main classes
- Attribute classes
- Filtering and SmartList
- SmartList
- Filtering
- Sorting
- Visualization
- Implementation Architecture
- Detailed documentation on each class, its methods and attributes
Quickstart
This API gives you access to most of the HyperCube features that you have from your browser. You can then: - load, edit, process, visualize your data in python before of after using a HyperCube feature. - write some scripts which will contain all your actions so that you can easily repeat them when you change your data source.
Connect
As in your web browser, everything starts with connecting to HyperCube with your credentials.
from HyperCube.python_api import API
api = API(username=your_username, password=your_password, url='https://your_url')
You can access the python API from a notebook launched via HyperCube. If this case api = API()
should work. You can also access a remote HyperCube server if you have the credentials.
Explore
As you may know, notebook or ipython console gives you some useful tools to explore the api.
By writing a dot at the end of an object and pressing tab you will see its methods and attributes. For example for
api.
+ 'TAB' you get:api.User api.Project api.VariableTag api.Variable api.XrayVariable api.Dataset api.KpiScore api.Kpi api.Xray api.Ruleset api.Correlation api.Prediction api.user
By writing an interrogation mark at the end of a class or a function and pressing 'ENTER' key, you will get some documentation. For example for
api.Project?
+ 'ENTER' you get:
Init signature: api.Project(self, **kwargs)
Docstring:
Class to retrieve or create a project.
From a Project instance, you will have an easy access to
its datasets, kpis, variable_tags and user
Project is derived from RestAbstractClass from which it shares methods.
See RestAbstractClass documentation for more information.
File: c:\github\hypercube\docs\pythonapiv3.0\python_api.py
Type: CreateSmartListMetaclass
Main objects to explore
The main classes that the api defines are: - Project
- Dataset
- Kpi
- Xray
- Ruleset
- User
- VariableTag
- Prediction
- Correlation
All of these classes derive from the same parent class and share the following main methods: - all
: to retrieve all objects (ex: projects = api.Project.all()
) - filter
: to retrieve a list of objects with criteria on its attributes (ex: projects = api.Project.filter(name='foo')
) - get
: to retrieve a unique object with criteria (ex: project = api.Project.filter(name='foo')
) - create
: to create a new object and eventually launch an algorithm (ex: projects = api.Project.create(name='foo')
) (For a dataset, create will upload it, for a xray, create will launch the algorithm) - get_or_raise
: to retrieve one object from HyperCube server matching criteria. An error is raised if many objects match the criteria or if no object is found. - get_or_create
: to retrieve one object from HyperCube server matching criteria. An error is raised only if many objects match the criteria. If no object is found, one will be created (using create
method) - save
: to save an object to HyperCube server. For now, users do not need to call this function. They shall call create
method instead - delete
: to delete the object on HyperCube server - update
: to update the object
Usage Examples
To get started with the python API of HyperCube, the best is to familiarize with the examples below. Note: For these examples we are assuming that our dataset are charged on a local mode (uploaded previously on the notebook) that's why the name local_filename it's used, if you want to access directly to your dataset you have to set the whole path on local_filename.
Playing with dataset retrieving projects
import os, sys
import importlib
import HyperCube.python_api
importlib.reload(HyperCube.python_api)
api = HyperCube.python_api.API(url='https://localhost')
#### Get connected to active project
project = api.Project.get_or_create(name='Titanic')
#### Adding Titanic dataset to the project
dataset = api.Dataset.get_or_create(project=project, name='Titanic', local_filename='Titanic.csv')
#### Show list of variable names containing in your dataset
variables = dataset.variables
print('Dataset variables:\n', variables.name)
#### Retrieve the list of projects that you have available
project = api.Project.all()
print(project)
#### Get connected to active project
project = api.Project.get_or_create(name='Titanic')
Playing with Dataset
import os, sys
import importlib
import HyperCube.python_api
importlib.reload(HyperCube.python_api)
api = HyperCube.python_api.API(url='https://localhost')
#### Get connected to active project
project = api.Project.get_or_create(name='Titanic')
#### Add Titanic dataset to the project
dataset = api.Dataset.get_or_create(project=project, name='Titanic', local_filename='Titanic.csv')
#### Show list of variable names
variables = dataset.variables
print('Dataset variables:\n', variables.name)
#### Show modalities of 'Survival Status' variable
print('\nModalities of "Survival_Status" variable:\n', variables.get(name='Survival_Status').modalities)
#### Tag all variables which name contains Ticket
variables_tagged_tickets = variables.filter(name__contains='Ticket').add_tags('Ticket')
print('\nVariables tagged tickets:\n', variables_tagged_tickets.name)
#### Tag all variables which concern identity
identity_variables_names = ['Name', 'Gender', 'Age', 'Sex']
variables_tagged_identity = variables.filter(name__in=identity_variables_names).add_tags('Identity')
print('\nVariables tagged Identity:\n', variables_tagged_identity.name)
#### Tag and ignore all variables which have more than 50% of missing value
variables_with_most_missing = variables.filter(ratio_missing__gt=0.5).add_tags('A lot missing').ignore()
print('\nVariables tagged "A lot missing":\n', variables_with_most_missing.name)
#### Show distribution of 'Survival_Status' variable
variables.get(name='Age').show()
Playing with X-ray
import os, sys
import importlib
import HyperCube.python_api
importlib.reload(HyperCube.python_api)
api = HyperCube.python_api.API(url='https://localhost')
#### Get connected to active project
project = api.Project.get_or_create(name='Titanic')
#### Add Titanic dataset to the project
dataset = api.Dataset.get_or_create(project=project, name='Titanic', local_filename='Titanic.csv')
#### Show list of variable names
print('Dataset variables:\n', dataset.variables.name)
#### Show modalities of 'Survival_Status' variable
print('\nModalities of "Survival_Status" variable:\n', dataset.variables.get(name='Survival_Status').modalities)
#### Performing x-ray
xray = api.Xray.get_or_create(name='Example SL2', dataset=dataset, target__name='Survival_Status', quantization_bins_nb=5)
#### Display variables that have been computed for x-ray
print('\nxray variables:\n', xray.variables.name)
#### select a variable to show and display its contrast rate
variable = xray.variables.get(name='Gender')
print('\nContraste Rate of {}:\n{}'.format(variable.name, variable.contrast_rate))
#### Sort Variables by contrast rate and tag the 5 first as 'important'
important_variables = xray.variables.sortby('contrast_rate', reverse=True)[:5].add_tags('important')
print('\n5 most important variables:\n', important_variables.name)
#### Ignore the 10 least 'important' variables
not_important_variables = xray.variables.sortby('contrast_rate')[:5].add_tags('not important').ignore()
print('\n5 least important variables:\n', not_important_variables.name)
#### Show xray visualisation for variableToShow
variable.show()
Playing with Ruleset
import os, sys
import importlib
import HyperCube.python_api
importlib.reload(HyperCube.python_api)
api = HyperCube.python_api.API(url='https://localhost')
#### Get connected to active project
project = api.Project.get_or_create(name='Titanic')
#### Add Titanic dataset to the project
dataset = api.Dataset.get_or_create(project=project, name='Titanic', local_filename='Titanic.csv')
#### Show list of variable names
print('Dataset variables:\n', dataset.variables.name)
#### Show modalities of 'Survival_Status' variable
print('\nModalities of "Survival_Status" variable:\n', dataset.variables.get(name='Survival_Status').modalities)
#### Perform Ruleset
ruleset = api.Ruleset.get_or_create(name='Example Ruleset', dataset=dataset, target__name='Survival_Status', target__modality='alive')
#### Print stats of ruleset
print('\nRuleset stats:')
print('Nb of rules: {}'.format(ruleset.count))
print('Coverage: {}'.format(ruleset.coverage))
print('Purity: {}'.format(ruleset.purity))
print('Nb of people in ruleset: {}'.format(ruleset.size))
print('Proportion of people in ruleset: {}%'.format(100 * ruleset.size / dataset.nb_rows))
#### Display variables that have been computed for ruleset
print('\nRuleset variables:\n', ruleset.variables.name)
#### select a variable to show and display its nb_of_appearance_in_rules
variable = ruleset.variables.get(name='Gender')
print('\nVariable "{}" appears {} times in rules'.format(variable.name, variable.nb_of_appearance_in_rules))
#### Sort Variables by nb of appearance in rules and tag the 5 first as 'important'
important_variables = ruleset.variables.sortby('nb_of_appearance_in_rules', reverse=True)[:5].add_tags('important')
print('\n5 most important variables:\n', important_variables.name)
#### Ignore the 10 least 'important' variables
not_important_variables = ruleset.variables.sortby('nb_of_appearance_in_rules')[:5].add_tags('not important').ignore()
print('\n5 least important variables:\n', not_important_variables.name)
Main API objects
Main classes
As indicated above, the main classes that the api defines are: - Project
- Dataset
- Kpi
- Xray
- Ruleset
- User
- VariableTag
- Prediction
- Correlation
They all derive from the same parent: RestAbstractClass
from which they share common methods. Below is a diagram showing each of theses classes in a box which indicates its attributes (upper part of the box) and its methods (below part of the box). In addition to their own methods, each class has the ones defined in RestAbstractClass
box.
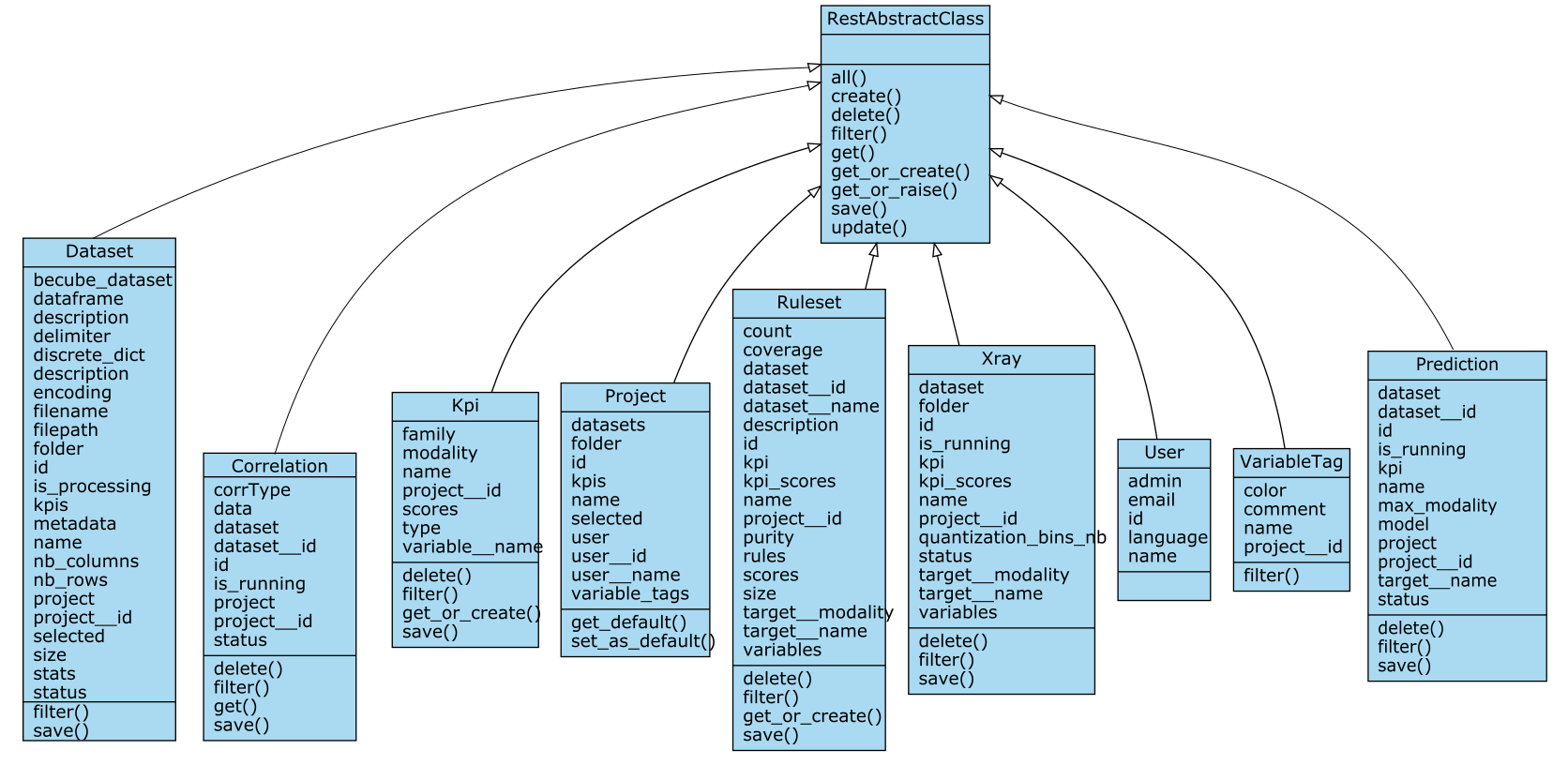
Shared methods
We give below the description of each shared method.
For more information on filtering (and thus on filter
, get
, get_or_raise
, get_or_create
methods), please see the Filtering section below.
For details on the arguments to pass to create
method, please see the specific documentation of the create
method of each class.
- all():
Retrieve all objects from HyperCube server - filter(**kwargs):
Retrieve list of objects from HyperCube server matching criteria - get(**kwargs):
Retrieve one object from HyperCube server matching criteria
If many objects match the criteria, an error will be raised - get_or_raise(**kwargs):
Retrieve one object from HyperCube server matching criteria
If many objects match the criteria, an error will be raised.
If no object is found, an error will also be raised. - get_or_create(**kwargs):
Retrieve one object from HyperCube server matching criteria
If many objects match the criteria, an error will be raised.
If no object is found, one will be created (usingcreate
method). - create(check_existence=True, **kwargs):
Create a new object and save it to HyperCube server (usingsave
method)
Some work may be processed on server side after save.
For example:- Data validation is performed after saving a dataset
- Simple Lift is performed after saving a simple lift
- Ruleset learning is performed after saving a ruleset
- save(self):
Save object to HyperCube server
For now, users do not need to call this function.
They shall callcreate
method instead - delete(self):
Delete the object on HyperCube server - update(self, obj=None):
Update the object attributes from obj
If obj is None, update from the object stored on HyperCube server.
Attributes of these main classes
Some attributes of these classes are instances or lists of instances of other of these classes. For example, given that project
is an instance of Project
: - project.datasets
is a list of instances of Dataset
- project.kpis
is a list of instances of Kpi
- project.user
is an instance of User
- project.variable_tags
is a list of instances of VariableTags
Whats more, some other attributes are instances or lists of instances of other classes (that we call attribute classes) among: - Variable
- XrayVariable
- RulesetVariable
- Rule
- KpiScore
For example, given that dataset
is an instance of Dataset
: - dataset.variables
is a list of instances of Variable
.
Attribute classes
Below is a diagram of these attribute classes which serve for the attributes of the main classes.
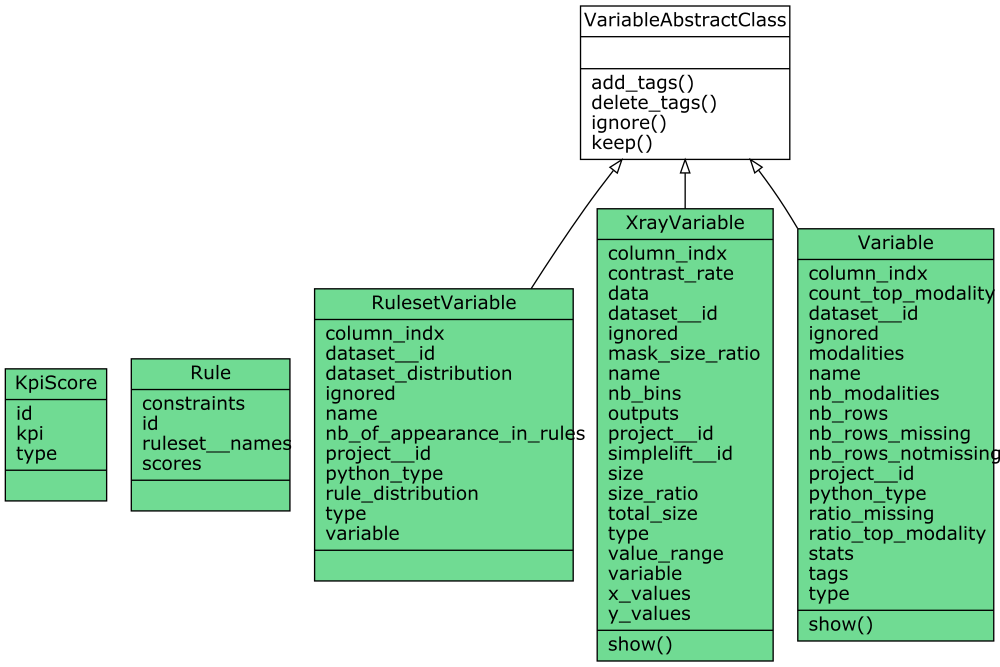
As you can see, Variable
, XrayVariable
and RulesetVariable
share some common methods.
Variable, XrayVariable, RulesetVariable shared methods
- add_tags(self, tags):
Add tags to this variabletags
may be:
- a tag name
- a list of tag names
- a VariableTag instance
- a list of VariableTag instances - delete_tags(self, tags):
Remove tags from this variabletags
may be:
- a tag name
- a list of tag names
- a VariableTag instance
- a list of VariableTag instances - ignore(self):
Ignore this variable - keep(self):
Do NOT ignore this variable
Filtering and SmartList
Before getting into details about filtering, we need to introduce you to SmartList
. If you are in rush or do not want to enter into details, we advise you to directly read the Usage Examples section to familiarize quickly.
SmartList
A SmartList
is nothing more than a standard python list
with extended functionalities.
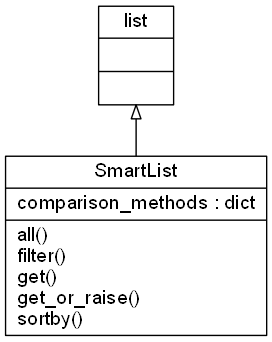
Put the table of SmartList methods documentation
When SmartList are used
Each of the 'main classes' and 'attribute classes' derive from the same JsonAbstractClass
which give them a custom SmartList
subclass (among other things) as shown in the diagram below.
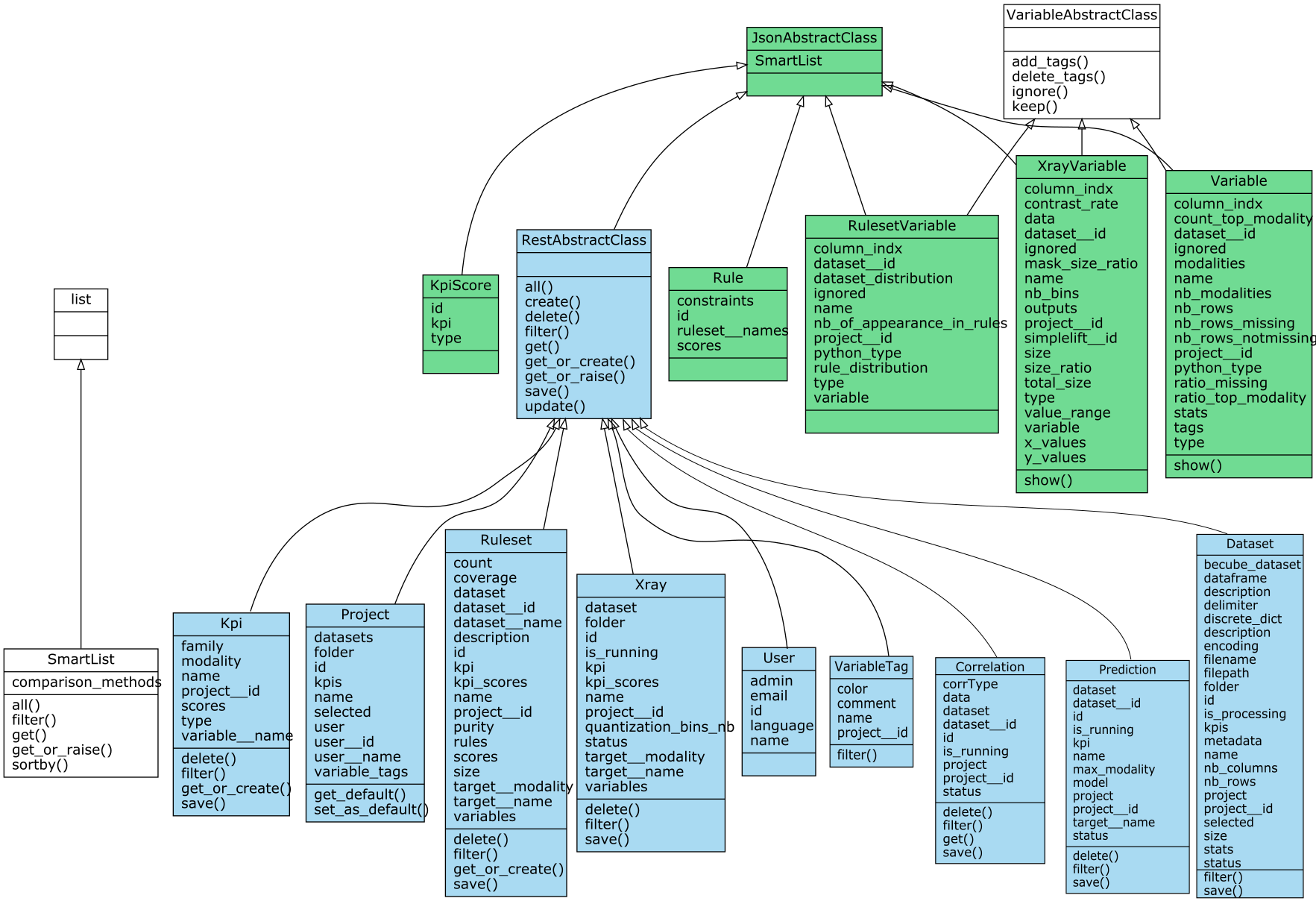
Show a diagram of Dataset.SmartList which derives from SmartList
Basically, SmartList
are used whenever the python_api returns a list of objects: - when we call the filter
method of a RestAbstractClass
, it returns a SmartList
of this RestAbstractClass
. For instance when you call Dataset.filter(name='foo')
it returns a Dataset.SmartList
. - when you get an attribute which is a list of objects, you actually retrieve a SmartList
of objects. For instance when you write Dataset.variables
it returns a Variable.SmartList
.
What SmartList are used for
SmartList
enables: - easy filtering (ex: retrieve the variables tagged 'important')(usage: important_variables = dataset.variables.filter(tags__name__contains='important')
) - easy sorting - retrieving the same attribute of all the objects as a list (ex: retrieve the names of all the projects)(usage: project_names = Project.all().name
) - calling a same method on all the objects (ex: add one tag to many variables)(usage: dataset.variables.add_tags('mytag')
)
Filtering
Filtering is invoked at different places - directly on a SmartList
- when you retrieve objects from server, that is to say in the following methods of a main class: filter
, get
, get_or_raise
, get_or_create
. Thus these methods eat the sames arguments as SmartList.filter
method.
Some properties
Filtering is done on attributes, or attributes of attributes, or at any level...
To filter on an attribute of an attribute: separate them by '__'- Comparison Operator
By default equality between attribute and given value is checked.
But many other comparison operator exist.
To use them add '__' followed by the operator code (ex: 'lte') as a suffix.
Operator codes:- eq (equal)
- gt (greater than)
- gte (greater than or equal)
- lt (lower than)
- lte (lower than or equal)
- in
- contains
- startswith
- endswith
Output
The filter function returns aSmartList
.
So anySmartList
method can be again applied (such assortby
).We tried to follow the well know syntax of Django ORM filtering.
Full Example
important_variables = dataset.variables.filter(tags__name__contains='important')
Here the tags attributes on a variable is a SmartList
of VariableTag
.
These VariableTag
have a name
attribute.
Thus, for one variable variable.tags.name
is a list of tag names.
We check that this list contains 'important'
How to know the attributes on which I can filter
You can filter on all the attributes given in the following diagram:
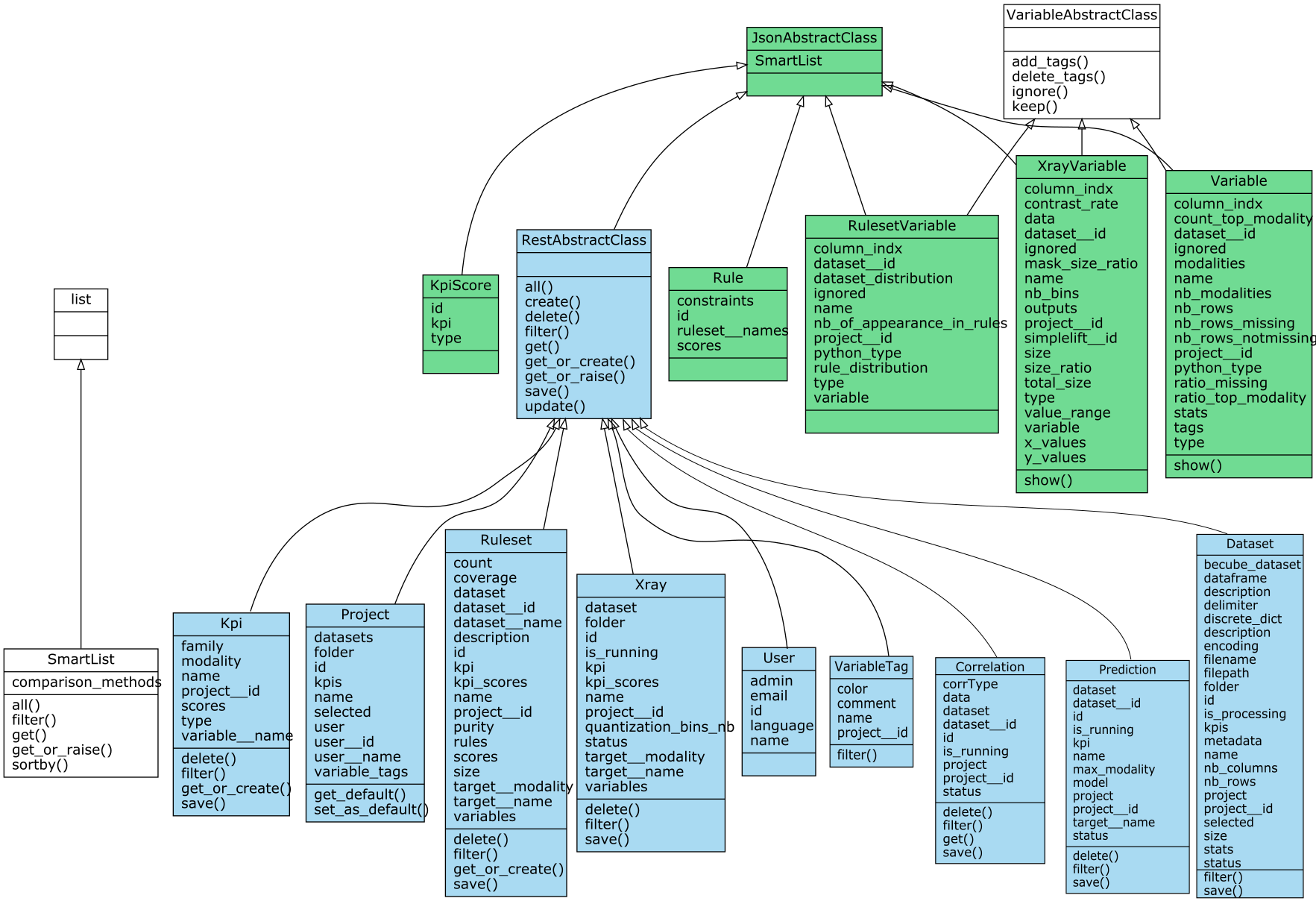
Sorting
Sorting objects in a SmartList
is really similar to filtering. We refer you to the Usage Examples.
Visualization
We aim to offer all the visualization you have on your browser directly into the notebook.
For now, we only offer a show
method on: - Variable
(show its distribution)(usage dataset.variables[0].show()
) - XrayVariable
(show the simple lift plot)(usage xray.variables[0].show()
).
Implementation Architecture
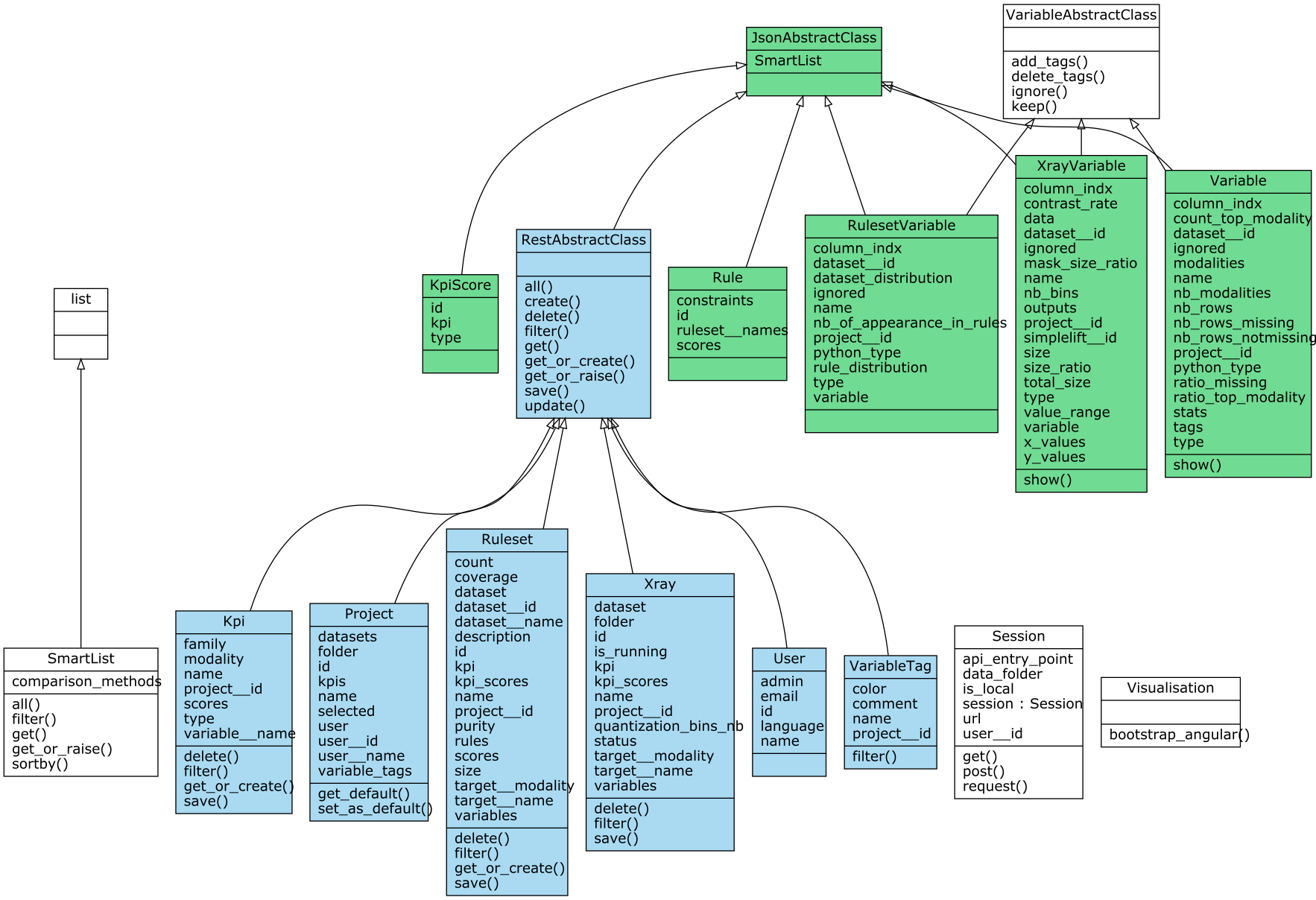